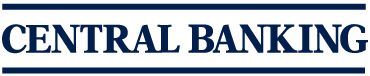
The untapped potential of transaction data

Covid-19 has highlighted the need for central banks to have more timely information, questioning whether high-frequency indicators are being used to their full potential.
The human brain is an amazing piece of machinery. A section of a brain’s cortex, one cubic millimetre in size – roughly the size of a grain of sand – can hold 2,000 terabytes of information. According to Konrad Kording, a neuroscientist at the University of Pennsylvania, the brain can churn through more information in 30 seconds than the Hubble Space Telescope could in 30 years.1
Even more astounding is the brain’s ability to forecast. When you ‘see’ something, only about 10% of the information comes from the optic nerve. Other parts of your brain have to deconstruct this information. For each visual input, it takes a minute amount of time – about one-fifth of a second – for the information to travel into the brain to be interpreted. To help us deal better with this fractional lag, the brain does an extraordinary thing: it continuously forecasts what the world will be like one-fifth of a second from now. That means we never see the world as it is at this very instant, but rather as it will be a fraction of a second in the future.2
Since the global financial crisis that began in 2007–08, interest in new macroeconomic forecasting tools, especially those based on monetary and financial information, has increased – the rapid rate of digitalisation means the data world has never been noisier. As a result, when the Covid-19 pandemic occurred, policy-makers had a wealth of statistics at their disposal that would not have been available a few years ago. In particular, central banks and financial supervisors realised the potential benefits of accessing very granular data on financial instruments such as loans and debt securities, as well as on the balance sheets of key financial institutions.
However, the pandemic also highlighted the need to go beyond standard datasets. Traditional indicators, such as inflation and unemployment data – which are commonly used by central banks when making monetary policy decisions – were not able to provide policy-makers with the information they needed, in the time they needed. Deputy managing director of the International Monetary Fund – Tao Zhang – noted long-standing data sources and surveys needed to be “replaced or revamped”.
“Traditional approaches to data collection, compilation and dissemination have had to be adjusted. In this context, key economic statistics may no longer tell us what we need to know with the same level of accuracy, and all users of official data need to keep this in mind,” he said.
The rising importance of high-frequency indicators
Speaking to Central Banking in January 2021, Karel Mertens, senior economist at the Federal Reserve Bank of Dallas, says high-frequency indicators have been on the radar of central banks for some time, but they have not looked to use them as they “typically do not add much to the precision of forecasts”.
However, during the pandemic, everything happened so much faster than expected, meaning the frequency of official data and traditional indicators used by central banks were not released in time for policy-makers to take action.
“It was quite clear we were going into a recession, and we could tell this was the case even without high-frequency indicators,” Mertens says. “But what was less clear, and this is where high-frequency data was useful, was in identifying the turning point.”
The Dallas Fed noticed in late April 2020 that the US economy was already starting to expand and, in effect, recover. “There was just no way you could tell that from any traditional sources,” Mertens says, adding that the Dallas Fed went on to revise its forecast for the year fairly significantly.
Data in action
In Israel, the central bank decided to leverage technology to gather different sources of real-time data to make quicker decisions. “In normal times, such information is too short-sighted to be helpful. But, with things developing rapidly, it has given critical insight into people’s habits,” says Andrew Abir, deputy governor of the Bank of Israel.
The central bank quickly established a new team and system to collect, organise and analyse new sources of real-time data. Many economic activities just stopped because there was nothing to record. Similarly, statistical models were unable to measure new activities that had replaced others.
According to Bruno Tissot and Barend De Beer, a case in point was the consumption of household spending and the related measurement of the Consumer Prices Index. “Spending on specific items went to almost zero due to Covid-19-related lockdowns and social distancing, while it surged for other items,” they said. “The difficulty for statisticians was to quickly adapt to these changing patterns, noting that the related weights are usually adjusted only progressively, often once a year.”
In Israel, the central bank’s new team was tasked with collecting data from internet sites and informal information sources within two days of a citizen’s activity. This included daily credit card purchases, which enabled the central bank to assess economic activity and trends.
Payment data
Payment data is an invaluable source of information for central banks. Recent studies have found that payment transactions – specifically data gathered from payment cards – can help with nowcasting and forecasting GDP and private consumption in the short term.
But very few central banks gather this data themselves; instead, the private sector – largely dominated by payment behemoths and new entrants into the bigtech arena – monopolise this arena. On February 10, 2021, Rory MacFarquar, senior vice-president of Mastercard, said the payments giant had gathered a lot of data on sales trends during the pandemic, which it then shared with policy-making institutions to understand trends right down to the municipal level.
The Bank of Israel, similarly, turned to Apple and Google during the pandemic. Both firms made the information public, while keeping individual identities private, to help authorities better respond to the global health crisis. For example, when traffic returned to commercial hubs, it signalled furloughs were coming to an end.
Some central banks, however, had a head start in using payments data when the pandemic struck. In Norway, the central bank has been gathering transaction data since 2017. Given the majority of the population uses digital payments – a recent survey from Norges Bank indicated only 4% of transactions were made with cash in the third quarter of 2020 – there is a profusion of information to play with.
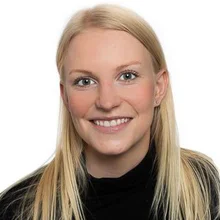
“When the pandemic broke out, we found it necessary to gather high-frequency real-time data,” explains Norges Bank economist Tuva Marie Fastbø. “Obtaining daily transaction data early in the pandemic has been vital to our nowcasts and understanding of the developments in household consumption.”
In March 2020, Norges Bank started to receive transaction data covering all domestic debit card transactions in physical terminals by Norwegian households on a daily frequency. “This card payments data is free of sampling errors and delays,” says Fastbø. In a working paper published by Norges Bank in 2020, economists concluded that debit card transaction data serves as an early and reliable indicator of household consumption in Norway.
Early hiccups
The increase in the proliferation and use of digital products and services increased the number of macro aggregates that central banks now need to observe. Technology challenges policy-makers’ understanding of price dynamics: is price stickiness still relevant for digital transactions? How do prices behave when the marginal cost of producing more is very small, even close to zero?
Implementing high-frequency data into forecasts, therefore, is no mean feat. In South Africa, research from the central bank revealed new statistical models still tended to overestimate GDP growth when used for nowcasting. This was, in part, due to domestic volatility, but the authors also noted there was a potential disconnect.
A working paper published by the South African Reserve Bank argues that changes in GDP need to be controlled in order to avoid future overestimation. “Greater care needs to be taken when drawing causal and inferential conclusion,” the authors say. If not, the relationship between the models’ “proxies of economic fundamentals and measured GDP” will continue to weaken.
In Norway, the central bank quickly realised that daily data is highly sensitive to seasonal and holiday variations and would require manual adjustment. “It also creates noise in the data,” Norges Bank senior adviser Kjersti Næss Torstensen explains.
Mertens also noted that most high-frequency indicators have short histories, which means it is difficult for central banks to know how they will behave through normal business cycles. “When recessionary dynamics were different from the ones we see now, much of this data suffers from inconsistent coverage,” he says.
Speaking to Central Banking in August 2020, Google’s chief economist Hal Varian noted that transactional data could be useful for foreceasting, as it tracks spending on and offline, but he also issued some words of caution: “There are weather effects, holiday effects and other high-frequency patterns that need to be taken into account when analysing the data.”
Not taking into account household consumption after the Norway’s national lockdown, economists at Norges Bank aggregated the data to weekly and monthly frequencies as a result. “Even though we are able to track unadjusted consumption figures from the national accounts, seasonal patterns are often adjusted manually at Statistics Norway,” says Torstensen.
In addition, the pandemic forced an even larger portion of the Norwegian population to use payment cards instead of cash. Card purchases have therefore tended to overestimate the increase in consumption. “To account for possible frequency mismatch, we have estimated Mixed Data Sampling regressions, which exploit the difference in sampling frequencies explicitly,” says Torstensen.
How the data is gathered is also an issue. Many high-frequency indicators are collected from different software and apps, which change over time as technology evolves. As a result, when there is a movement in the data, central banks cannot be sure whether it is picking up a signal about the economy or if it is related to the fact the data collection process has changed, Mertens said.
Collaborative effort
Central banks’ changing their approach to data has had a ripple effect across other agencies when it comes to decision-making. The Bank of England (BoE) has signalled it will provide the Office for National Statistics (ONS) with credit and debit card transaction data for the first time. The aim is to better inform policy-makers about how people have spent their money during the pandemic.
Currently, the BoE tracks daily credit and debit card payments through the Clearing House Automated Payment System (Chaps), connecting to around 100 major retailers. The BoE will anonymise and aggregate the data before sharing with the UK’s national statistical institute for publication.
While ONS retail sales figures provide monthly estimates on what consumers are buying and how much they are spending on items in shops and online, the Chaps data will provide greater granular detail. The new data will also add information on spending related to social activities.
“This transaction data represents a big step forward in our ability to see how purchasing habits have changed during all the stages of the pandemic so far and, going forward, will continue to provide valuable insights,” says David Matthewson, head of faster indicators at the ONS.
The ONS has broken the data down into four consumption categories: staples (food, communication and utilities), delayable (clothing, household goods and vehicles), work-related (public transport and fuel) and social (travel, entertainment and hospitality). Each sector in the series is weighted according to its relative share of annual UK household consumption in Q4 2019.
Since the end of January, the ONS has published the data on a weekly basis as part of its fast indicator series, providing access to experimental data on the impact of Covid-19 using rapid response surveys.
Learning from within
The pandemic has highlighted the need for high-frequency indicators to be utilised more by central banks.
A recent paper from the Bank of Italy used a large-scale payment dataset to predict Italian GDP and its main components; the contribution of payment system flows to improving forecasting accuracy the authors conclude is “non-negligible”. Moreover, the timeliness of the data improves nowcasting accuracy.
Mertens’ says credit card data in particular will be incredibly valuable for policy-making in the future. “In January, we have already seen a big uptick in credit and debit card spending in comparison to weak consumption data from December. We won’t get our January consumption data for quite some time, but the high-frequency data has picked this up already,” he says.
But there is still a lot for central bankers to learn. One drawback of high-frequency data is that it only tells a story about the very short term. Ben Broadbent, deputy governor at the BoE, says high-frequency data has given policy-makers “a pretty good steer” on consumer spending, and helped inform the BoE’s forecasts for the next two quarters. But “it doesn’t resolve the big question we’ve been spending some time talking about, which is: ‘What about the medium term?’”
So perhaps central banks should look to build new data frameworks that mimic the human brain – a tool to provide both short-term and long-term forecasts. According to the World Economic Forum, by imitating the way neurons function, both energy and real costs can be drastically decreased for complex computational tasks including the mining of data in noisy environments.
Notes
1. Alison Abbott (2013), Neuroscience: Solving the brain, Nature 499, pp. 272–274.
2. Douwe Draaisma (2017), Perception: Our useful inability to see reality, Nature 544, p. 296.
Only users who have a paid subscription or are part of a corporate subscription are able to print or copy content.
To access these options, along with all other subscription benefits, please contact info@centralbanking.com or view our subscription options here: http://subscriptions.centralbanking.com/subscribe
You are currently unable to print this content. Please contact info@centralbanking.com to find out more.
You are currently unable to copy this content. Please contact info@centralbanking.com to find out more.
Copyright Infopro Digital Limited. All rights reserved.
As outlined in our terms and conditions, https://www.infopro-digital.com/terms-and-conditions/subscriptions/ (point 2.4), printing is limited to a single copy.
If you would like to purchase additional rights please email info@centralbanking.com
Copyright Infopro Digital Limited. All rights reserved.
You may share this content using our article tools. As outlined in our terms and conditions, https://www.infopro-digital.com/terms-and-conditions/subscriptions/ (clause 2.4), an Authorised User may only make one copy of the materials for their own personal use. You must also comply with the restrictions in clause 2.5.
If you would like to purchase additional rights please email info@centralbanking.com