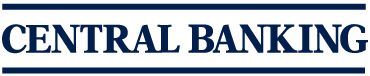
The changing data landscape: Part 2
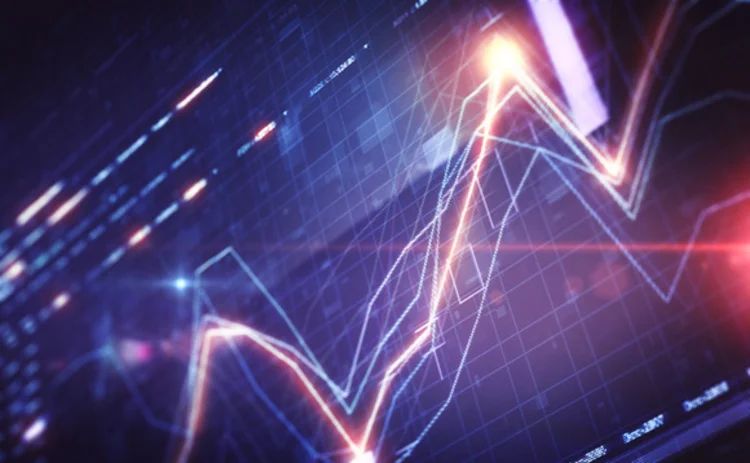
The way central banks think about data is changing. As the world becomes more digital, there is an ever-growing data tool from which central banks and regulators can draw information about the economy. But old legacy systems are preventing this information from being drawn into decision-making processes.
In many countries, firms are still using outdated reporting tools to provide central banks with critical information about their liquidity positions and solvency. Meanwhile, data concerning everyday economic activities – including retail transactions – is not being used to its full advantage.
However, there is some progress being made. The use of artificial intelligence (AI) and machine learning is being deployed and collaboration with bigtech firms to source data is increasing.
In the second of this two-part series, Central Banking speaks to three policy-makers on the evolving data landscape and what central banks must do to adapt to a data-driven policy world.
What are the biggest data challenges your institution faces when making economic policy decisions, and what are the current solutions?
Juan José Ospina: The main challenge is how to collect data in a formal, systematic and timely manner that allows the central bank to better track the economy in real time. Assessing the economy in real time allows a central bank to better understand the shocks and how they affect the economy. And I think the Covid-19 crisis has highlighted the need to look into different data.
When the pandemic broke out, the Central Bank of Colombia looked at its traditional indicators and also those of statistical agencies that publish real-time indicators of how the economy is doing – but we didn’t know everything.
For example, commercial banks have real-time information on the transactions people make using credit and debit cards, which allows them to track real time in real-time spending trends within each sector and region. That is something we do not have the ability to do, but would like to. If a central bank does not have a good sense of how the economy is doing, then the policy recommendations made may not be appropriate. Thankfully, when we asked the commercial banks to share this information with us, they did, but there is nothing in the legislation that mandates them to do this; we could get to a stage when sharing data is not a priority. When receiving data from different sources, there are additional challenges relating to format. Each institution publishes its data in a different way, there is no standard that makes it difficult to compare and transmit information quickly.
Philip Abradu-Otoo: Data fragmentation is one of the biggest challenge for us. There is also the challenge of merging the various data types the central bank receives to form a big, quality and reliable database that can be easily accessible on various platforms to facilitate research and decision-making.
Other challenges focus on the ability to rapidly access, effectively manage, process in a timely manner, and analyse growing volumes of economic, financial, supervisory and statistical data/information. It is also worth noting the limitations in human and IT resources that allow ideal real-time access and efficient processing of data/information.
Occasionally, technical hitches during data transmission present a challenge. Problems with file formats sent to the central bank also remain an issue. Where providers of this data are focused on individual staff rather than institutionally, risks associated with data transmission could occur. When these individuals become unavailable for any reason, data submission gaps emerge and could act as a drag on the time lapse needed for policy decision-making.
Howard Chang: There are shared data challenges and common predicaments for the entire central bank community, including limited data sources, timeliness, inefficient transmission, varied formats, data fragmentation, granularity, colossal volume, accessibility, confidentiality and regulations.
Numerous novel approaches have been deployed to address these issues. They include the introduction of additional data sources, simplifying reporting procedures with the help of regtech solutions, and building unified data lakes.
However, despite these cutting-edge advancements, policy-makers still find it difficult to garner a clear understanding of the state of the economy, not to mention the more granular forces at play in the national economy. The tools and methods mentioned above are indeed admirable, yet fall short of an effective solution.
At TCSA, we have developed an innovative methodology to resolve these data challenges once and for all by tracking real-time transactions of the entire society via nationwide payment terminals. Akin to “the blood in the bloodstream for an economy,” this granular data will yield a birds’-eye view of national economic dynamics with the highest resolution. Worthy of attention is that this precise picture of the macroeconomic landscape will not be built on needlessly complex IT or data technologies but, instead, based on a stunningly simple concept: data standardisation on a national level.
Data plays a key role in informing central banks’ decisions. How does your institution currently collect data? What kind of data? And how do you organise these datasets with other sources of financial data (fiscal, for example?)
Juan José Ospina: We collect data in very different ways, including directly from analysts, businesses and financial institutions. But it is likely different datasets are collected by different departments of the central banks. For example, the financial stability department might conduct a survey while economists looking at inflation might ask for different data. Data is also collected through regulatory reporting. In Colombia, we require all foreign exchange transactions to be reported to us so we know how many US dollars are going in and out of the country; it is also helpful to know what types of transactions are being made in foreign currencies. And, finally, we collect data through co-ordination with other government agencies. In these instances, we tend to share information to a schedule – daily or monthly. It really depends on what data agreement we have in place. For example, the Consumer Confidence Index is created by a private think-tank, but we have an agreement with them whereby we basically pay them to produce the survey.
In terms of the types of data we collect, the majority is microdata from financial institutions, aggregated by sector. We also collect data through surveys, which are similar to the US Federal Reserve’s Beige Book. And we also collect data from other companies like Datastream and Bloomberg, which also aggregate financial statistics. Aggregating them is where is gets complicated. We have several systems that capture all of this information, and they do not always talk to one another – so we have a system that communicates with them and extracts information. We also have systems that organise our datasets according to the needs of the end-user. For example, a system might collect an entire set of data, but an analyst might only want data from a certain institution or over a specific time period. There are also certain permissions for accessing certain datasets as well. From this data we can produce economic analysis, as well as our own statistics and indicators.

Philip Abradu-Otoo: The Bank of Ghana has well laid-down structures for collecting primary and secondary data. These are executed through surveys, submission of prudential reports by banks and other international institutions, and other secondary data sources.
Regarding surveys, questionnaires are sent to respondents via email. This has its own limitations at times and has proven not to be an effective data-gathering tool as the email responses are often not fully completed. This has meant that staff have often had to physically visit respondents to ensure full completion of the questionnaires before processing.
We also have a straight-through process via which the central bank receives data. For example, banking supervision reports on the deposit money banks and other supervised non-bank financial institutions would be submitted in this way.
Organisation of fiscal data is very important given the role of government in the economy, and given the unique role of the central bank as the banker to the government. The behaviour of government is measured through its revenue mobilisation efforts and spending behaviour. As a result, gathering and organising information on governmental operations is important to inform the entire policy formulation process, on the monetary and fiscal policy sides.
Howard Chang: The common approach of data collection by central banks today still lies in the gathering of traditional data sources through manual surveys across various entities, and quarterly or annual reports from financial institutions. Some have begun digging into secondary data sources across governmental departments or purchasing additional internet-based data from the private sector.
In the words of Ousmène Mandeng, a visiting fellow at the London School of Economics: “It surprises me that, with so much data, central banks are not using the data at their doorsteps.” In fact, central banks already have direct and unfettered access to the most logical and ubiquitous sources of information regarding all human economic activities – monetary transactional data. When aggregated, this primary data provides a real-time snapshot of the entire economy.
Central banks are already halfway there in terms of solving the issue of inadequate available data sources. Therefore, the fastest, easiest and cheapest upgrade of national-level data collection and data processing has to start from, and be based on, existing central bank data systems. It is the most viable path and the lowest-hanging fruit.
With a simple algorithmic software add-on grafted onto all existing payment terminals, these data can be captured into one standardised metadata package that encompasses all dimensions of socioeconomic activities. This means pieces of data will no longer be scattered into different silos, removing the risk of fragmentation and the trouble of data cleansing further down the road.
How should central banks approach the issue of data fragmentation?
Juan José Ospina: At the moment, if you ask banks for certain datasets, they give it to you in whatever format is easiest for them. Most of these firms use different platforms and software to aggregate and collect data, it is never one size fits all. In some cases, they do not even organise it. So pulling information from a number of institutions and then comparing it is major challenge.
In this instance, we would look to standardise data at the source. This gives you control over what is then handed over. But there is also the issue of data repetition – where numerous institutions ask for the same data, sometimes different departments asking for the same set of data. You end up asking consumers or firms for the same information over and over again, and this is not sustainable. For me, fragmentation has to start with solving issues within the central bank first.
I would ask institutions to send as much data as they can, and then pull it together in a single central database where it can be standardised and accessed by different departments. There will be challenges that remain – a centralised system is going to need to be interoperable with those of the firms reporting the data, and vice versa. Some departments may also need certain datasets in different formats to other departments, but it would be a start. You would at least have the primary data and can then apply any kind of aggregation or computation needed.
Philip Abradu-Otoo: To keep up with the evolving data demand, the Bank of Ghana has developed a shared internal platform to enable different departments to access data resources within the institution.
The statistics office within the research department collates, processes and manages data from both the primary and secondary sources and then sends the information to another unit for further data consistency checking. A unit within the research department of the bank receives data from these various data sources, check for consistencies with other key macroeconomic variables and initiates an approval process within the institution before it is consumed by the policy-makers and for subsequent publication and dissemination via the bank’s main communication channels.
Within the bank, there are also clear internal processes that exist to ensure data interrogation before such data is presented to management for policy discourse and decision-making. Such processes of intense data interrogation (structured and unstructured) unearths inconsistencies in the data, allowing for fragmented data to be coherently repaired before being used for analytical and policy work.
Howard Chang: To address data fragmentation, most central banks choose to extract data from various silos into a unified data lake, which ultimately form what has become known as the hub-and-spoke architecture. It is a powerful approach, but an alternative and more efficient path can be found by fundamentally reconstructing current data infrastructure.
Rather than following the conventional logic, where attempts to bridge data from various silos at huge cost into one centralised data lake after the silos are formed, our new proposition capitalises on the fact that all pieces of monetary transactional data can already be synchronised and organised into a standardised metastructure at the point of collection. This allows for data integration on a real-time basis at a large scale, while operating at a cost that is one-hundredth of traditional data infrastructure.
Furthermore, under a homogeneous format, these metadata packages are ready to be accessed and computed by various departments for various usages. They act as the basic building block for all data architecture, laying the foundation for an extremely cost-effective computing mechanism for data on the national scale. They can be added, subtracted and calculated quickly and cheaply, allowing policy-makers to know at a glance how much water is consumed in each province, how many roads are needed in each city or how much of what resource is consumed for which purposes.
As the world becomes more digital, a larger volume of consumer data will be collected by firms and, in some instances, by regulators. What are the data protection implications of using consumer data to better inform central bank policy-making?
Juan José Ospina: The main challenge is actually ensuring each institution you are collecting data from is compliant with data privacy regulation. Sometimes consumers provide data on the understanding is not going to be shared, even if the institution asking for the data has its own policy in place or a good reputation.
Once you have the microdata, there is also the added issue of storing it in a secure manner. The volume of data financial institutions gather has never been greater, and so new technology has been developed to gather, aggregate and store this data. With new technology comes new risk, and ensuring a data breach does not occur is a priority for central banks. One solution that has emerged for the private sector is cloud technology. It is harder for central banks to use these systems because they are often owned by third parties that have introduced an additional layer or additional risks; some central banks have developed their own cloud technology, but this is not common. So getting the information is hard, storing it is also difficult. One could argue that while the digital age has revolutionised financial services, it has also made it more challenging.
Philip Abradu-Otoo: The central bank is increasingly developing requirements to ensure data protection. I think your question is basically trying to assess how we strike that fine balance between our regulatory functions, which requires gathering larger volumes of data and respecting data protection and data rights issues.
Already there are rules and regulations that protect the data we collect from depositors, lenders, borrowers and other counterparties, and how this data is used for policy. As regulators, we have all signed up to some form of confidentiality agreement with our partner bodies, we follow confidentiality rules in line with International Organization for Standardization requirements, there are data restrictions on staff regarding the sensitivity of data that can be assessed and, in instances where banks have to provide consumer data to parent companies, they are required to seek clearance from the Bank of Ghana and data protection agencies.
These rules and regulations have evolved over time and, as the economy undergoes a digitisation phase, I see these rules and regulations also changing with the exigencies of time. Looking ahead, I see these rules and regulations bordering on consumer data, and other forms of big data will also be required to evolve with constant fine-tuning to help better inform policy. All put together, central banks must remain watchful of these evolving trends while building on the necessary mechanisms to protect the data that is received from consumers.

Howard Chang: The heated debate on data protection could be examined from several angles.
First, data sharing and privacy are not in binary opposition because privacy can be ensured through technology. For example: anonymisation, output checking, physical controls, authorisation,and cloud technology. User data can be fully collected, but the rights to access and use it should be clearly defined within systemic sets of policies and regulations.
Second, the tension and balance between technological breakthroughs and individual freedom can be revisited from a different perspective where data-empowered informed decisions actually bring citizens the truest sense of freedom. For example, in Sweden citizens opt to use electronic means over traditional payment methods. As a result, the authorities have collected massive amounts of data for analysis, policy-making and research purposes.
Third, there would be an element of data supervision. To prevent the potential abuse of power and violation of data confidentiality. It serves as an enabler for government entities to share with the public its decision-making rationale and logic underneath.
The status quo is that private data has never been genuinely owned by citizens, but instead largely controlled by tech giants that have been monopolising and monetising consumer data. Yet, whether market-oriented business behaviours can ensure data protection begs a number of questions. We believe that establishing a centralised data powerhouse is the safest way to return that data ownership to individuals and prevent data breaches.
How do you see a central bank’s role evolving within a data-driven policy-making space?
Juan José Ospina: At the moment, there is no need to centralise data collection. Every company has its own agenda when it comes to data, and they should be free to collect, aggregate and store it whichever way they see fit. It is not a central bank’s role to govern these things.
But we also have to move with the times. There are now more sources of data available to central banks – more high-frequency indicators that can give us a real-time snapshot of how the economy is doing. At the moment, many central banks operate in a very ‘constrained’ environment, which means, realistically, all the data has to be analysed ‘yesterday’. I think the ability to use new real-time data is out there – we know it is because some firms are already using it. I do see a future where central banks are trying to grab more of that information, and not only to make more timely policy decisions. During the Covid-19 pandemic central banks were able to respond quickly and appropriately, but it would be good to regularly monitor certain metrics to better understand the dynamics of the economy. It is important to understand how shocks impact different regions, income groups, social groups and more. Currently, doing this type of research takes a lot of time, but for policy-making purposes that time may not be available. We need to ensure we have the data so we can make decisions more quickly.
Philip Abradu-Otoo: As the digital space evolves, the Bank of Ghana is working to provide timely economic and financial data that can be accessed with ease by all stakeholders to facilitate enhanced and quick decision-making processes.
Banks now submit data online, which has helped greatly during the pandemic era; ultimately, the central bank’s supervisory role has been enhanced through this approach to data submission. The Bank of Ghana is also developing requirements for the financial institutions to improve data management and technological innovations to reduce the inherent risk associated with technological innovations.
Howard Chang: TCSA agrees central bankers should be the leaders of a data-empowered future. That is why we have built an open-access data platforms upon central banks’ existing cloud architectures for data-sharing and maximised accessibility.
Granular data captured through the lens of monetary transactions will project the clearest view of the economy with supply and demand, inputs and outputs of each industry for decision-making. Furthermore, the highly compatible data can power machine learning forecasting models and numerous other toolkits, such as AI and natural language processing, for in-depth analysis. The management of data in this way takes the mystery out of monetary policy-making and enables central banks to make precise adjustments based on accurate information.
Furthermore, the centralisation of national economic data will lay the solid groundwork for a hard value anchor for national currencies by realigning currency prices with real economic values. Currencies can be pegged to each country’s actual economic strength – as quantified by real-time data. As a result, inflation could be better managed; internationally, the credibility and status of a national currency can be improved for global trade.
Finally, the platform also makes data easily accessible for the private sector to spur further innovations. In this sense, the platform is similar to the dual cloud environment piloted by leading central banks with dashboards to query data. Data resources and services could then be transformed into a steady source of fiscal revenues or income for central banks, for example, in the form of data tax.
Interviewees
Juan José Ospina, chief officer for monetary policy and economic information, Central Bank of Colombia
Philip Abradu-Otoo, director of research, Bank of Ghana
Howard Chang, vice-president of global affairs, Thousand Cities Strategic Algorithms (TCSA)
This article will feature in a forthcoming report entitled ‘Improved central bank data management in a digital age’. Part 1 of this interview series can be found here.
Only users who have a paid subscription or are part of a corporate subscription are able to print or copy content.
To access these options, along with all other subscription benefits, please contact info@centralbanking.com or view our subscription options here: http://subscriptions.centralbanking.com/subscribe
You are currently unable to print this content. Please contact info@centralbanking.com to find out more.
You are currently unable to copy this content. Please contact info@centralbanking.com to find out more.
Copyright Infopro Digital Limited. All rights reserved.
As outlined in our terms and conditions, https://www.infopro-digital.com/terms-and-conditions/subscriptions/ (point 2.4), printing is limited to a single copy.
If you would like to purchase additional rights please email info@centralbanking.com
Copyright Infopro Digital Limited. All rights reserved.
You may share this content using our article tools. As outlined in our terms and conditions, https://www.infopro-digital.com/terms-and-conditions/subscriptions/ (clause 2.4), an Authorised User may only make one copy of the materials for their own personal use. You must also comply with the restrictions in clause 2.5.
If you would like to purchase additional rights please email info@centralbanking.com